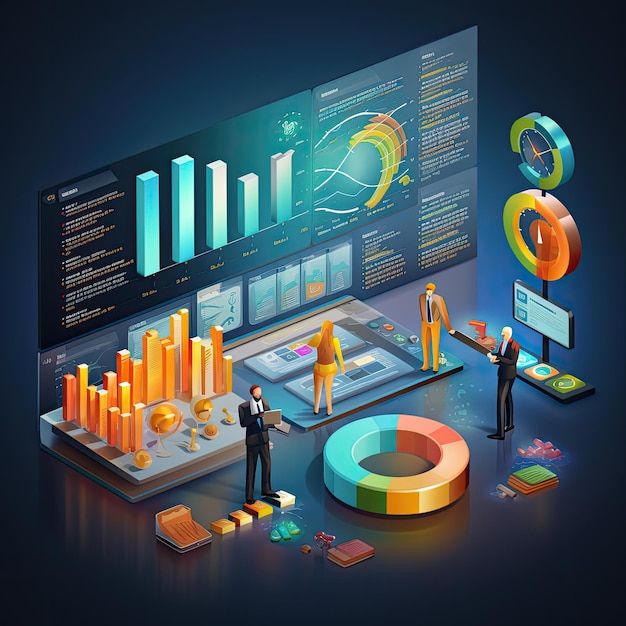
Analytics Process Model and Data Sources for data collection
What is Data Visualization ?
- Data Visualization is the process of presenting information or data in a visual format, like graphs, charts, or maps.
- Instead of looking at rows and columns of numbers, you're turning that data into pictures that are easier for our brains to understand.
- These visual representations help us quickly grasp patterns, trends, and relationships in the data without having to analyze each individual number.
Analytical Process Model
- An analytical process model is a structured way of breaking down and
- organizing the steps involved in analyzing data to gain insights or make decisions.
- It's like a roadmap that guides you through the entire process from start to finish.
Data Sources
- Data sources are places or locations where you can find information or data. They're like the starting points for collecting data
- Data sources can come in various forms, such as databases, surveys, websites, etc and even people's observations or experiences.
- Websites: Online platforms where data can be gathered from user interactions, such as clicks or searches.
- Data sources are crucial because they provide the raw material for analysis and decision-making.
- Without reliable data sources, it's challenging to gain insights or solve problems effectively.
- When selecting data sources, it's essential to consider factors like the relevance, and accessibility of the data.
Data Selection
- Determine the specific goals or questions you want to address with your analysis.
- Knowing your objectives will guide you in selecting the most relevant data.
- Establish criteria for selecting data based on its relevance and usefulness for your objectives.
- Consider factors like time period, geographic location, and data quality.
- Identify where the data is coming from. It could be internal sources like databases or spreadsheets within your organization, or external sources like public datasets or surveys.
- Gather the datasets or variables that meet your criteria and align with your objectives. Be selective and focus on acquiring the most pertinent data to avoid unnecessary complexity.
- Assess the quality of the selected data to ensure its accuracy, completeness, and consistency. Check for errors, missing values, and anomalies that could affect the reliability of your analysis.
Data Cleaning
- Look for mistakes or inconsistencies in the data, such as missing values, duplicate entries, or incorrect spellings.
- This step involves carefully examining the dataset to spot any issues that could affect the accuracy of your analysis.
- Decide how to deal with missing values in the dataset.
- You can either remove rows with missing values, fill in the missing values with a placeholder (like zero or the average),
- or use statistical methods to estimate missing values based on other data.
- Check for and remove any replicating records in the data.
- Duplicates can skew your analysis by inflating certain values or misleadingly representing the data.
- Removing them ensures that each observation is unique and contributes only once to the analysis.
- For example, dates should be in the same format (e.g., MM/DD/YYYY or YYYY-MM-DD),
- and categorical variables should use the same naming conventions.
Data Transformation
- Data transformation involves rearranging or reorganizing the data to make it more useful for analysis.
- This might include changing the layout of the data, combining multiple datasets, or splitting one dataset into smaller parts.
- Before analysis, data often needs to be cleaned and formatted. This step involves removing errors, inconsistencies,
- or irrelevant information from the dataset. It also includes standardizing data formats and ensuring consistency across different variables.
- Sometimes, it's necessary to create new variables or metrics from existing data to better answer analytical questions.
- This could involve performing calculations or deriving new insights from the existing dataset.
- In data transformation, normalization and standardization are techniques used to scale and adjust the range of values within the dataset.
Analytical Patterns
- Analytical patterns involve identifying recurring structures or trends within the data.
- For example, you might notice a pattern where sales of a certain product increase during specific seasons or holidays.
- Analytical patterns often involve applying specific techniques or methods to analyze the data.
- This could include statistical methods like regression analysis, clustering, or time series analysis.
- By recognizing patterns, you can generate insights or conclusions about the data.
- For instance, identifying a pattern of increased website traffic during weekends might lead to the insight that weekends are peak times for online shopping.
- Analytical patterns can sometimes help predict future outcomes based on past data.
Interpretation and Evaluation
- Interpretation involves making sense of the findings from your analysis.
- You look at the patterns and trends in the data to understand what they mean in the context of your objectives or questions.
Drawing Conclusions
- Based on your understanding of the results, you draw conclusions about the situation or problem you're analyzing.
- You determine what the data is telling you and what implications it has for decision-making.
Assessing Significance
- Evaluation involves assessing the significance or importance of your findings.
- You consider factors like the size of the effect, the reliability of the data, and the relevance to your objectives.
Making Recommendations
- If your conclusions have practical implications, you may make recommendations for action based on your analysis.
- These recommendations could be changes in strategy, policy, or behavior informed by the insights gained from the data.
Reflecting on Process
- Finally, you reflect on the analytical process itself.
- You consider the strengths and limitations of your analysis, including any challenges encountered or areas for improvement.
- This reflection helps you learn from the experience and refine your approach for future analyses.
Analytical parts in different profiles
- Analytical parts in different profiles refers to the components or elements of data analysis that are applicable across various industries, or job roles.
- These analytical parts are fundamental aspects of the data analysis process that can be tailored and applied to specific contexts or domains.
- Analytical parts encompass the common steps and techniques used in data analysis, such as data collection, cleaning, analysis, interpretation, and visualization,
- but they are adapted and utilized differently depending on the particular field or professional role.
Marketing Analyst
- Analyzing customer demographic data to identify target market segments.
- Evaluating the performance of marketing campaigns based on key performance indicators (KPIs) such as conversion rates and return on investment (ROI).
- Interpreting social media engagement metrics to assess brand awareness and customer sentiment.
- Using predictive modeling techniques to forecast sales trends and optimize marketing strategies.
- Creating data visualizations, such as charts and dashboards, to communicate insights to stakeholders.
Financial Analyst
- Analyzing historical financial data, such as balance sheets and income statements, to assess company performance.
- Evaluating investment opportunities by conducting risk assessments and financial modeling.
- Interpreting economic indicators and market trends to forecast future financial conditions.
- Performing variance analysis to compare actual financial results with budgeted or forecasted figures.
- Generating financial reports and presentations to support decision-making by executives and investors.
Human Resources (HR) Analyst
- Analyzing employee turnover data to identify factors influencing employee retention and develop retention strategies.
- Evaluating workforce demographics and diversity metrics to assess workforce representation and equity.
- Interpreting employee performance metrics, such as performance ratings and productivity measures, to inform talent management decisions.
- Conducting compensation analysis to ensure pay equity and market competitiveness.
- Creating workforce planning models and scenarios to forecast staffing needs and address talent gaps.
Operations Analyst
- Analyzing production data to identify bottlenecks and optimize manufacturing processes.
- Evaluating supply chain performance metrics, such as inventory turnover and order fulfillment rates, to improve efficiency.
- Interpreting customer service data to identify opportunities for improving service quality and customer satisfaction.
- Conducting time series analysis to forecast demand and optimize inventory levels.
- Generating operational reports and performance dashboards to monitor key performance indicators (KPIs) and track progress towards operational goals.
Healthcare Data Analyst
- Analyzing patient healthcare records to identify trends and patterns in medical diagnoses and treatments.
- Evaluating the effectiveness of healthcare interventions and treatment protocols using clinical outcome measures.
- Interpreting healthcare utilization data to identify opportunities for cost savings and resource optimization.
- Conducting epidemiological studies to track disease prevalence and monitor public health trends.
- Creating data visualizations, such as heatmaps and geographic information system (GIS) maps, to visualize healthcare disparities and access to services.
Conclusion
We have explored analytics Process Model, Analytics part in different profiles, Analytical Model Requirements and Data Sources for data collection